HYPE: Chat GPT and AI Take on the Insurance Industry! Insurtechs Vs. Incumbents
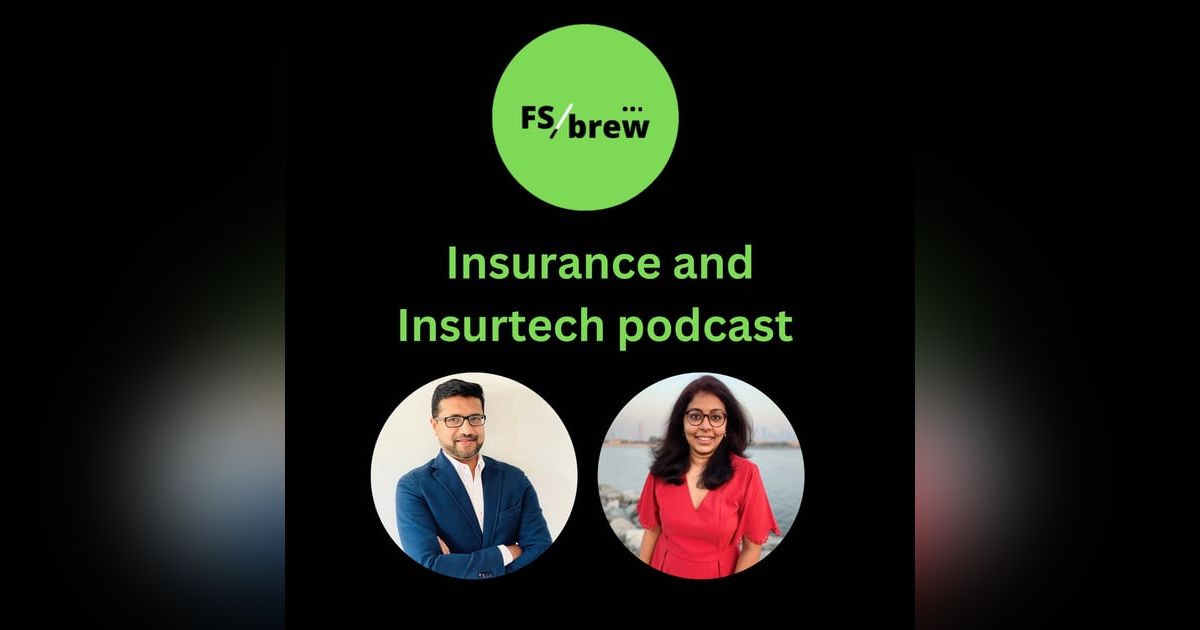
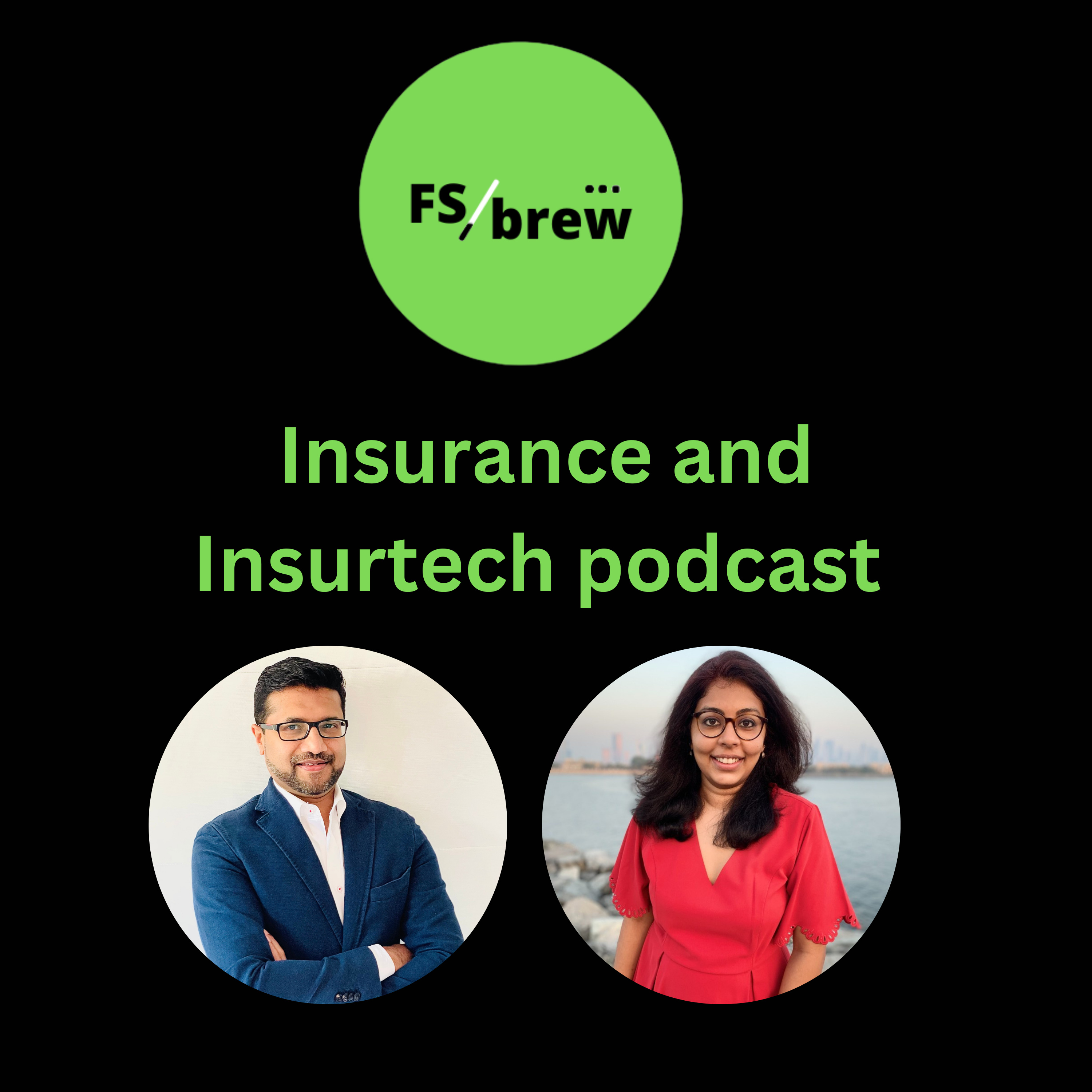
Chat GPT and AI in Insurance
# Welcome to the latest episode of FS Brew Hype
## **Chat GPT and AI in Insurance**
Insurance is a complex and data-driven industry, and Chat GPT and AI are two emerging technologies that have the potential to revolutionize the way insurance is done.
Chat GPT is a large language model that can be used to create chatbots that can answer customer questions, process claims, and even underwrite policies. AI can be used to analyze data and identify patterns that can help insurers make better decisions about risk and pricing.
Together, Chat GPT and AI can help insurers improve customer service, reduce costs, and increase efficiency. They can also help insurers to better understand and manage risk, which can lead to more accurate pricing and more profitable underwriting.
## Here are some specific examples of how Chat GPT and AI are being used in insurance:
- **Underwriting:** AI can be used to automate the underwriting process, which can free up underwriters to focus on more complex cases. AI can be used to analyze data and identify patterns that can help insurers make better decisions about risk.
- **Claims processing:** AI can be used to automate claims processing, which can speed up the claims process and improve customer satisfaction. AI can be used to identify fraudulent claims, which can help insurers to reduce costs.
- **Customer service:** Chat GPT can be used to create chatbots that can answer customer questions 24/7. This can help insurers to improve customer service and reduce costs.
The use of Chat GPT and AI in insurance is still in its early stages, but the potential benefits are significant.
## Here are some additional thoughts on the potential impact of Chat GPT and AI in insurance:
- **Personalization:** AI can be used to personalize insurance products and services. For example, insurers could use Chat GPT to create chatbots that can tailor insurance recommendations to each individual customer's needs.
- **Prevention:** AI can be used to prevent insurance fraud. For example, insurers could use AI to analyze claims data and identify patterns that may indicate fraudulent activity.
- **Innovation:** AI can be used to innovate in the insurance industry. For example, insurers could use AI create new products and services that meet the needs of a changing customer base.
Overall, the potential benefits of Chat GPT and AI in insurance are significant. These technologies have the potential to revolutionize the insurance industry and make it more efficient, effective, and customer-friendly.
*Tags: #AI #insurtech #chatgpt*
--- Send in a voice message: https://podcasters.spotify.com/pod/show/fsbrew/message
[00:00:06] Renjit: welcome to a very special episode of FS Brew Hype. So this is where we take up a topic that is really trending. And to be honest, we are a little slow to get onto this, but we wanna take our time for you guys to make sure that we give you a deep down into how Chat GPT and AI are completely revolutionizing a lot of things, including insurance.
[00:00:27] Vidya: Absolutely, you were pretty bang on. And the fact that generally us, and also the insurance industry, slowly doing a waiting watch in terms of what this trend can do. For us as an industry, and we are so excited to present a number of possibilities that's out there from an AI and very specifically from a Chat GPT perspective.
[00:00:47] Essentially, there is any way a need to innovate quite substantially when it comes to insurance industry, and I think injecting AI is just going to be imminent. We've seen that across many industries, and it's only going to come [00:01:00] to the insurance sector pretty much anytime now.
[00:01:03] Renjit: I think we, we all have been lamenting the lack of innovation in this space. Long been industry is long recognized for its complex process. Reliance on paperwork, slow response times. Not everybody, but most of us. So traditional insurance face these challenges like high operational costs. Let's say lack of one-on-one, personalized underwriting, inefficiencies in underwriting claims, and so on and so forth.
[00:01:29] And also very limited use of data and analytics, to be honest. And that's the foundation for ai, right? So if you're not using data analytics Forget about advanced models and algorithms like generative AI of the likes of chat G P T uses, right? So obviously customers are dissatisfied. NPS scores are not so great.
[00:01:49] So that's a great foundation for why AI should be used. And this is, I'm not just talking about the region. This is a global picture.
[00:01:56] Vidya: Absolutely. And you know what is exciting about the [00:02:00] whole piece around AI and an extension of that is Chat GPT is the ability to. Really make it work for you in the region.
[00:02:08] So in the geography that you are in, typically all the models and just the way the structure has been, has always been adapted from different countries, advanced mature countries, especially on the actuarial side, on within the insurance sector. With this, we hope you get deeper into the data side of things, which is very much localized, and you're able to build up a pattern and you're able to apply a lot more.
[00:02:30] Closer to what this geography needs.
[00:02:33] Renjit: So just a little bit of a few words on AI and Chat GPT. Obviously AI is work on, AI has been going on for almost decades. And really from forties, but only now with the arrival of high computing power and memory going, the cost of memory going down, et cetera. And obviously advances in the algorithm development itself, we are seeing tremendous movement in the sophistication of the AI model.
[00:02:57] So that's one thing. And just to put things in perspective, Chat [00:03:00] GPT users, what is known as an LLM or a large language model, and they've been trained on billions of basically websites, things that are available on the internet. And it's developed by OpenAI originally set up by Elon Musk and Sam Altman, and now obviously Elon Musk has moved away and there is drama there.
[00:03:17] We might not cover that drama in this episode. You never know With us, anything can happen. Absolutely. So some general areas of where AI and Chat GPT could be applied. Yeah. Obviously we know that underwriting is very generic these days. It could be personalized with the availability of data.
[00:03:38] What about, what are your thoughts on claims and other areas? Customer service?
[00:03:43] Vidya: No, absolutely. So just all the underwriting piece itself, actuarial sciences. Definitely quite advanced and whatnot. There is different models out there already in this market, like the G LM and all of those. So if you add that on to, with even more sophistication that comes in with this sort of tool, [00:04:00] the possibility feels quite endless on the product side, on the pricing side.
[00:04:04] So you can go a lot more micro, you can go a lot more macro if you want, and therefore, you know that sort of possibility is there and like you rightly touched upon. But you've got possibilities on claims, you're on customer service. On just product innovation and distribution. Let's talk a little bit about applications of AI and machine learning in the underwriting and pricing space.
[00:04:28] A little bit of an old time example, old new example is root insurance. Yeah. It's US-based auto insurance company, pretty well known that uses AI and machine learning to offer personalized pricing based on driving habits, right? Individual driving habits. So it's telematics data collected from. Customer, smartphone.
[00:04:47] So the algos that Root deploys, assesses the driving behavior such as breaking acceleration, cornering, so on and so forth. And you can potentially offer personalized premiums. You can reward [00:05:00] safe drivers and penalize the bad drivers will potentially not even come to you because of the pricing. So that's definitely an example, a positive example of AI being used.
[00:05:10] So that's there. In probably the life insurance space. I think there's a company called Ladder Life, again, US based, and they use underwriting and machine learning to analyze customer data, age, health, lifestyle factors. Again, the premiums are at stake and we have seen variations of this in the health space, like vitality, which is a very old example in terms of if you walk more, you get lower premiums and so forth.
[00:05:38] We have WellX doing. Not exactly AI, but a similar kind of, I'm assuming it's a straightforward model. I think
[00:05:44] they're on the, yeah, they're on the path of it. They have the AI capability plugged in. I think they just have building that conversation that needs to go in, the data that needs to go into ultimately create their own models.
[00:05:54] And you actually said the life insurance piece is one of those areas that can really benefit from [00:06:00] the whole AI piece. I think when we spoke with the likes of from HAYAH insurance, they were also talking about how they are plugging in the whole AI model, AI piece into the stickiness of a product within, in a certain customer persona person X probability to buy a product Y would be more versus product Z and so on, so forth.
[00:06:19] So they use AI in AI's intelligence to build that sort of knowledge. Piece as well. Yeah, so you have some examples locally also available and of course, yeah. You mentioned good ones on the international side of things
[00:06:31] Renjit: as well. There's another interesting one that I recently picked up. It's called Understory.
[00:06:35] I don't know if you've heard about this. This is a US again, US based example. I'm afraid all my examples are from the US. This is basically on property risks, so they're using weather sensors and they have a platform so they can analyze very localized hyper-local weather data. To determine the impact of weather events on properties.
[00:06:56] So you could potentially create personalized pricing on very [00:07:00] location specific risks. So for example, for arguments sake, in Al Barsha you would have a particular property rate, and in Jumeira you could have a different rate if the weather systems were so complex as they are in other geographies.
[00:07:12] Vidya: So the whole geography piece reminds me of a company based in India called The Plum, which provides insurance packages through corporate.
[00:07:21] And ultimately what they want to do is to have Chat GPT and AI used in terms of location and give the customer live data in terms of where is the nearest hospital or a clinic that need to, they need to go to and use that whole intelligence on where they are to provide them immediate information and feedback and help and support.
[00:07:41] Okay,
[00:07:42] Renjit: so claims that's another. Pretty critical area, and we know that the industry is plagued by those three alphabets, FWA, Fraud waste, and I forget the a I'm sure you'll tell me later on. Abuse. That's the, yes. There are a couple [00:08:00] of companies in this space as well. One is called FRISS, I think this is based in Europe.
[00:08:04] It's not very clear from their website, but a number of clients there. So essentially it's all about using AI to detect fraud and claims patterns, which are looking out of the ordinary, which is a good application for, basically, it's an anomaly detection application for AI, which is a standard application, so fraud detection and risk mitigation.
[00:08:24] So their AI-driven platform uses machine learning algos to analyze claims data, identify patterns or anomalies that may indicate fraudulent activities. So by integrating with the existing insurer system. So I think it plugs into the core system of an insurer and they can process claims in real time. So the advantage is using this, you can actually allow simple cases to go through and claims be paid and treat complex cases differently, which is an issue, right?
[00:08:56] We always say claims take a lot, takes a long time, [00:09:00] and we always wonder if there's a way of triaging, which does not. Lead to fraud thoughts.
[00:09:07] Vidya: Yeah, absolutely. And just on the trust itself, is this across all product lines? Yeah. It
[00:09:11] Renjit: looks like it's across both personal and complex risks. Commercial risk as
[00:09:15] Vidya: well.
[00:09:15] Yeah, it's fascinating like you said, when you have to process claims at scale, it becomes pretty complex for insurers when you don't have certain patterns read, and therefore you cannot process it as fast as you want to. Especially when it comes to even the health insurance side of things. I think there's a lot of possibility of integrating AI into this and really transforming the whole claims turnaround time.
[00:09:38] And a couple of startups that I picked up on was Tracable. I think it's specific. Towards motor insurance and it tracks claims fraud using AI technology. But that said, Renjit, there are a couple of, I think InsureTechs here who are looking to do similar things as well on the motor side of things.
[00:09:55] Because there's so much of data that RTA now has connected over a period of [00:10:00]time, you're able to create really strong patterns and no fraud detection. Process claims and whatnot, and there is a company here and forgetting their name as of now what into that piece of work. And also as part of some of the conversations I've had with some of the leaders here, I think on the health side, on the claims side, it's a very fragmented process in the UAE because you have a broker system, then you have for the copy.
[00:10:27] Yeah, typically take the health, they'll have a system, so it's a very fragmented from system to system. And then you'll have a finance person who will sign off the claims amount and health claims potentially, and especially life claims, potentially. It's a very depressing thing, and at this point is when you know there's a moment of truth, the customer has to feel like, this is a piece of work I don't have to deal with and I just wanna get off it.
[00:10:50] So it is a very strong case for AI to come in.
[00:10:54] Renjit: Another one I saw was Shift Technologies. I think this pretty well known. We have a [00:11:00] platform called Force. Basically again, AI and machine learning to analyze claims, look at fraud behavior, so it, it considers various factors. claimant relationships, claim histories, geospatial data to create a risk score and it flags the claim as potentially fraudulent and investigators get a detailed explanation of the risk factors.
[00:11:23] Supporting evidence. So this potentially moves the claims faster and streamline the investigation resolution is faster, cheaper in terms of cost. So here's a great example of where AI can be used to minimize losses, maximize customer trust, improve operational efficiency. That's something not to be afraid of.
[00:11:44] From a claims department's point of view, we have this fear of AI taking over our jobs. It's just making us better.
[00:11:51] Vidya: Yeah, absolutely. And then when you look at the Chat GPT specifically in this case, you can use their API services to build virtual [00:12:00] assistance. Therefore, you can give set support versus having a certain office hours, which is now the current scenario.
[00:12:07] So again, there is obviously the customer service angle as well that can be added here. Yeah,
[00:12:12] Renjit: absolutely. And that's a good point. Yeah. And if you give access to internal APIs, some of these claims can be handled at midnight when your claims team is not really working with the help of Chat GPT. So how do you think we can use AI and for distribution?
[00:12:28] Vidya: Yeah, so this is an area I'm really excited about because just the sheer possibilities looks quite exciting on the distribution side, and we can also come to the whole product innovation side in a bit on the distribution side, I think some of the examples that we have seen in the Asian markets is how Plum has used again, and we go back to the example of Plum.
[00:12:46] They want to be able to reach out to their customers better and consolidate their whole ability to have that customer with them for a longer time. One of the pain points that they have identified is the customers don't really understand the policy wording as well [00:13:00] as they should be, or they just don't know how to navigate through.
[00:13:03] So they have used Chat GPT specifically integrated into their system and called it policy GPT, and that helps them. That helps the customer to understand policies and the wordings and the whole thing better in a way, conversational rate, which is what ideally every insurance company should be aiming for is.
[00:13:22] Renjit: Yeah, sure. I think another example that I picked up of, I think from France is Slice Labs. This is an Insurtech that offers on demand and usage-based insurance solutions, and they partner with Axa XL, which is obviously a global insurance and reinsurance company based in raised out of France. So what happens is Axa XL utilizes slices insurance cloud services to create customized on-demand insurance products for commercial clients.
[00:13:50] This shows that even in the complex commercial space, you can use AI and by getting together with partners like this, your [00:14:00] distribution is becoming more efficient. You can come out with some innovative products that match the industry and so on and so forth. So that's, that I thought was a decent example of how distribution is being used in the personal side.
[00:14:13] I think one of the examples is probably PolicyGenius. This is a US-based Insurtech, very much like our insurance market, and it's really an online marketplace, but then it uses AI to match customers with suitable products, so from a range of providers, so the customer doesn't have to go through the whole list of products and choose.
[00:14:34] AI says, Hey, this makes it slightly better for you based on the data that we have from a past customer. So that's an interesting way. It's a recommendation engine, what Amazon uses, basically. So it's not rocket science, but it's something useful.
[00:14:48] Vidya: No, which is great. Which is working beautifully for Amazon.
[00:14:51] We all know the cognitive load is something that works against the customer so unnecessary. If you give too many it work, so may as well give them what sticks to them and they [00:15:00] don't have to think too much and just go on with the journey and sell. But on the product side itself, Renjit, and let's maybe a little deeper, what are you excited about on the product innovation side when it, and when it comes to a AI application and there therefore the possibilities itself.
[00:15:17] Renjit: I think one thing that pops to mind is the example of Toffee insurance in India, right? Yeah. Yeah. So this is a Microinsurance product company, as we probably know, and it's really very simple products, affordable, customizable, like for example, dengue insurance, right?
[00:15:34] It just covers dengue and it's a problem in India. Great. Just give that Or bicycle insurance. There's lots of bicycles in Southeast Asia. Just for that. And the idea is if it's make it digital and really easy to claim, then the customers see the value of insurance and then they can graduate to other products such as motor home and stuff like that when they [00:16:00] act, go up the affluence ladder.
[00:16:02] So those kind of things excite me because it is really tackling the problem of under insurance.
[00:16:09] Vidya: Yeah, absolutely. Say going really deeper into what the customer wants real time as well. And I think we saw that with YAS. Insurance. One of the guys that, you know, one of the startups that we spoke with.
[00:16:20] I've done a fabulous job of using that sort of intelligence, AI and different other tools, of course, to give really good real time products, and that's also one of my favorites, the whole possibilities of coming up with insurance that works for you in this geography.
[00:16:35] Renjit: Alright. We spoke about all the good things of AI.
[00:16:37] What are the risks and issues to consider?
[00:16:39] Vidya: Yeah, so there are a couple of challenges and ethical issues obviously globally being spoken about, and just the whole fear of AI taking over the whole human race itself is, The larger fear, but specific to insurance itself, I think there is probability of biases coming into these algorithms, biases around race, gender [00:17:00] demographics, other demographics itself, and therefore your pricing models.
[00:17:03] Everything is still towards those biases. As we all know it is, algorithm is built on what we feed in. So we, we obviously have biased model, so how does that get negated into, or fed into these models? So that's one big thing. And anything else that you want to talk about Renjit? Yeah,
[00:17:19] Renjit: I think also the underlying data that's used to construct these models, right?
[00:17:24] So if my data is being used to create a model, then am I going to be, is my data going to be exposed to a number of insurance companies, to tech players who are going to construct the model? Am I going to be remunerated for it? Is my data going to be handled safely? I think all those issues come into play, so I think insurers have to take care of.
[00:17:45] Anonymization the usual standards of high security for data protection and all that. So that's definitely a given. And just moving on from the risks point of view, clearly this represents a significant job loss potential [00:18:00] for people who are doing operational work in insurance, in the insurance sector. So that is something to think about.
[00:18:08] How will. These people be retrained will be, will they be able to use AI as an assistant or not? I think in the immediate term, I don't see immediate job losses or anything, but definitely people who get onto the AI bandwagon and learn to use it will probably see that they're jumping up in the career ladder.
[00:18:28] Thoughts Vidya?
[00:18:29] Vidya: No, absolutely. So as you likely said, as of now that the argument is still me, that human loop in the, especially on the customer side of conversations with the between the call center team and the customers, AI cannot simply just take on all the load currently. Yeah, at some point the pivot will be there and it's just obviously for everyone of us to pick up that talent or pick up that skill set on long-term, and it's just not applicable just for us as I insurers or within the insurance ecosystem.
[00:18:58] I think it is just [00:19:00] generally there across our sectors itself. That's something that, and everyone should be mindful but yeah, that said, it's a very exciting topic. It's, it is definitely coming. It is up to every insurer to really gear up and see how we can really apply all of that into their current scenario.
[00:19:15] Chat GPT currently gives a lot of those API services. How do we use that to make it quite exciting for the customer? Because the customer currently is using for other things as well, right? So they are used to Chat GPT now they're used to using then LinkedIn content or whatever the details that you are doing with Chat GPT
[00:19:31] so they're getting used to it, so why not get them used to it with your organization as well? Yeah, absolutely.
[00:19:38] Renjit: I think that's a good point. Start experimenting with it as organizations get, get that free api. Also, there's actually a paid API access. Get that the pro version just cost $20 per month.
[00:19:49] That's worth investing in to play around and understand where you can apply. It's a brave new world. It's time to exploit it. This is probably a very good point to bring the HYPE episode to a close. [00:20:00]
[00:20:00] Vidya: Absolutely. So we really hope you enjoyed this episode. We certainly enjoyed putting this together. It's a fascinating area.
[00:20:06] It's an area we are getting to learn as well. Tune in and let us know how you or what you thought of this
[00:20:11] Renjit: episode. Goodbye. Absolutely. I will be very happy to get the feedback.
[00:20:15]